Retrieving and Highlighting Action with Spatiotemporal Reference
In this paper, we present a framework that jointly retrieves and spatiotemporally highlights actions in videos by enhancing current deep cross-modal retrieval methods. Our work takes on the novel task of action highlighting, which visualizes where and when actions occur in an untrimmed video setting. Leveraging weak supervision from annotated captions, our framework acquires spatiotemporal relevance maps and generates local embeddings which relate to the nouns and verbs in captions.
Moment-Sentence Grounding from Temporal Action Proposal
Deep learning in Vision and Language, which is one of a challenging task in multi-modal learning, is gaining more attention these days.
In this paper, we tackle with temporal moment retrieval. Given an untrimmed video and a description query, temporal moment retrieval aims to localize the temporal segment within the video that best describes the textual query. Our approach is based on mainly two stage models. First, temporal proposals are obtained by using the existing temporal action proposal method. Second, the best proposal is predicted by the similarity score between visual features and linguistic features.
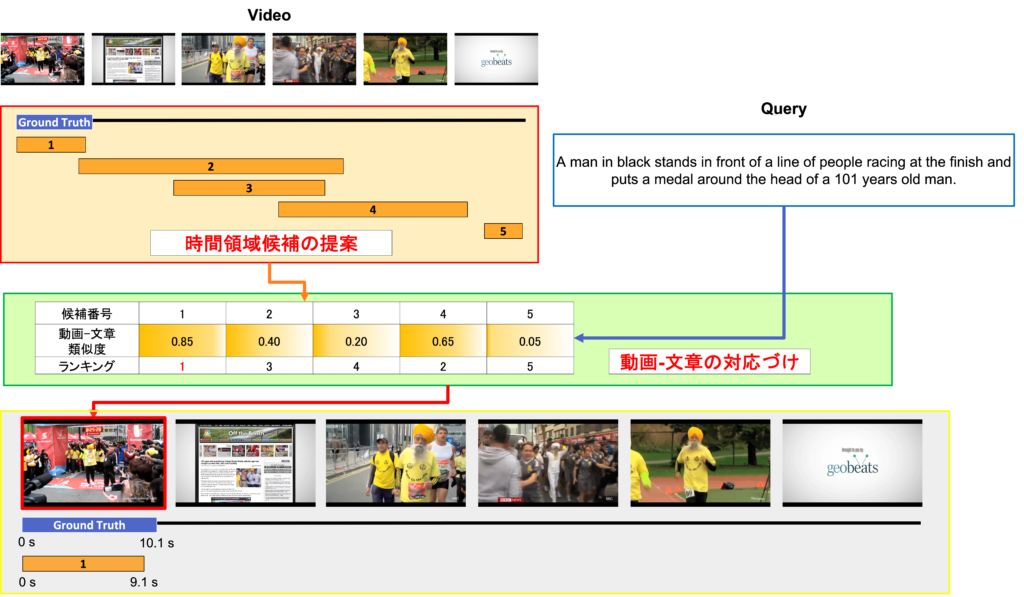