Optical flow estimation by in-vehicle event camera
We propose regularization specialized to in-vehicle camera scenes, which utilize characteristics relating to vehicle motion characteristics and focus of expansion (FOE) for optical flow estimation using event cameras. FOE is defined as the intersection of the translation axis of the camera and the image plane. The optical flow has the feature of becoming radial from the FOE when the component due to rotation is excluded from the optical flow of the surrounding environmental due to the motion of the vehicle itself. The proposed regularization restricts the direction of the optical flow using this feature. The usefulness of this regularization was demonstrated by evaluating the rotation parameters estimated during the method.
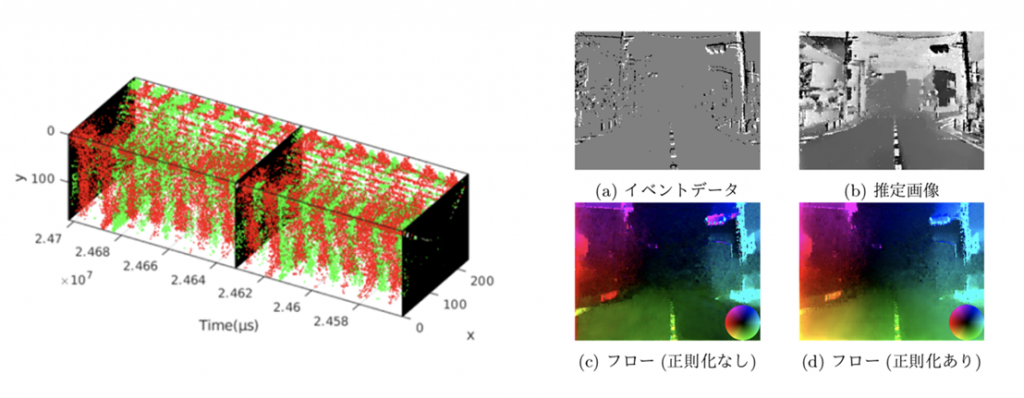
Robust QR-code recognition by using event camera
QR codes are widely used on production lines in factory automation. However, there is a problem that blurring occurs due to lighting conditions and the speed of the belt conveyor. Against this problem, event cameras asynchronously capture changes in luminance for each pixel and have excellent advantages such as high temporal resolution and high dynamic range. In this research, we proposed a method of estimating QR code robustly from event data by optimizing in QR code space which is more restricted than image space.
Scoliosis screening by estimation of spinal column alignment from moire topographic images of the back
In this study, we propose a method to calculate the Cobb angle and VR angle necessary for fully automatic scoliosis screening, using input of moire topographic images of the back of subjects without X-ray exposure. Using a moire images and X-ray images, we propose a method of estimating spinal column alignment coordinates with high precision and a method to automatically calculate Cobb angle and VR angle from spinal column alignment information from just a moire image by CNN learning with feature point coordinates of a spinal column extracted from an X-ray image by a physician as teacher data. We demonstrated the effectiveness of the proposed method on an independently constructed dataset. Currently, we are investigating a method to estimate 3D spinal column alignment from 3D scan data of the back.
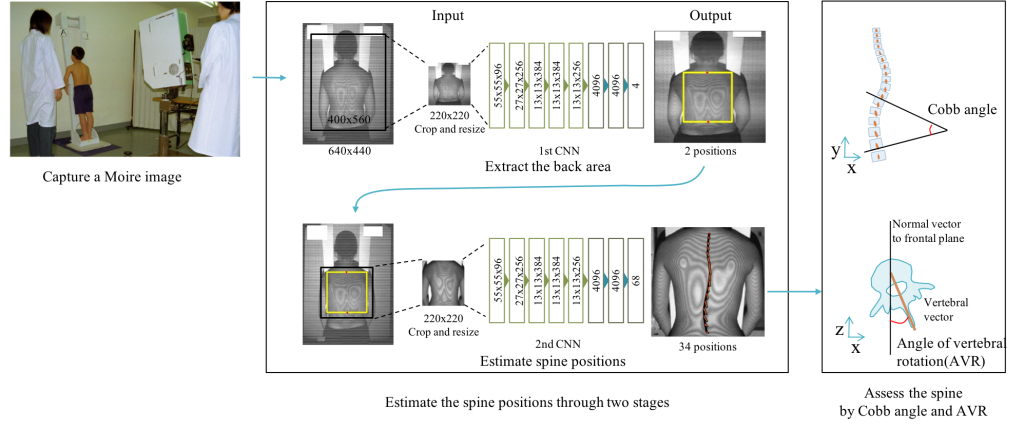
Application of Change Detection via Convolutional Neural Networks in Remote Sensing
Analysis of remote sensing imagery plays an increasingly vital role in the environment and climate monitoring, especially in detecting and managing changes in the environment. Since obtaining satellite imagery or aerial imagery are getting more comfortable in recent years, changes in landscape due to disaster are highly in demand. In this paper, we propose automatic landscape change detection especially landslide and flood detection by implementing convolutional neural network (CNN) in extracting the feature more effectively. CNN is robust to shadow, able to obtain the characteristic of disaster adequately and most importantly able to overcome misdetection or misjudgment by operators, which will affect the effectiveness of disaster relief. The neural network consists of 2 phases: training phase and testing phase. We created our own training data patches of pre-disaster and post-disaster from Google Earth Aerial Imagery, which we are currently focusing on two countries: Japan and Thailand. Each disaster’s training data set consists of 50000 patches, and all patches are trained in CNN to extract region where the disaster occurred without delay. The results show the accuracy of our system in around 80%-90% of both disaster detections. Based on the promising results, the proposed method may assist in our understanding of the role of deep learning in disaster detection.
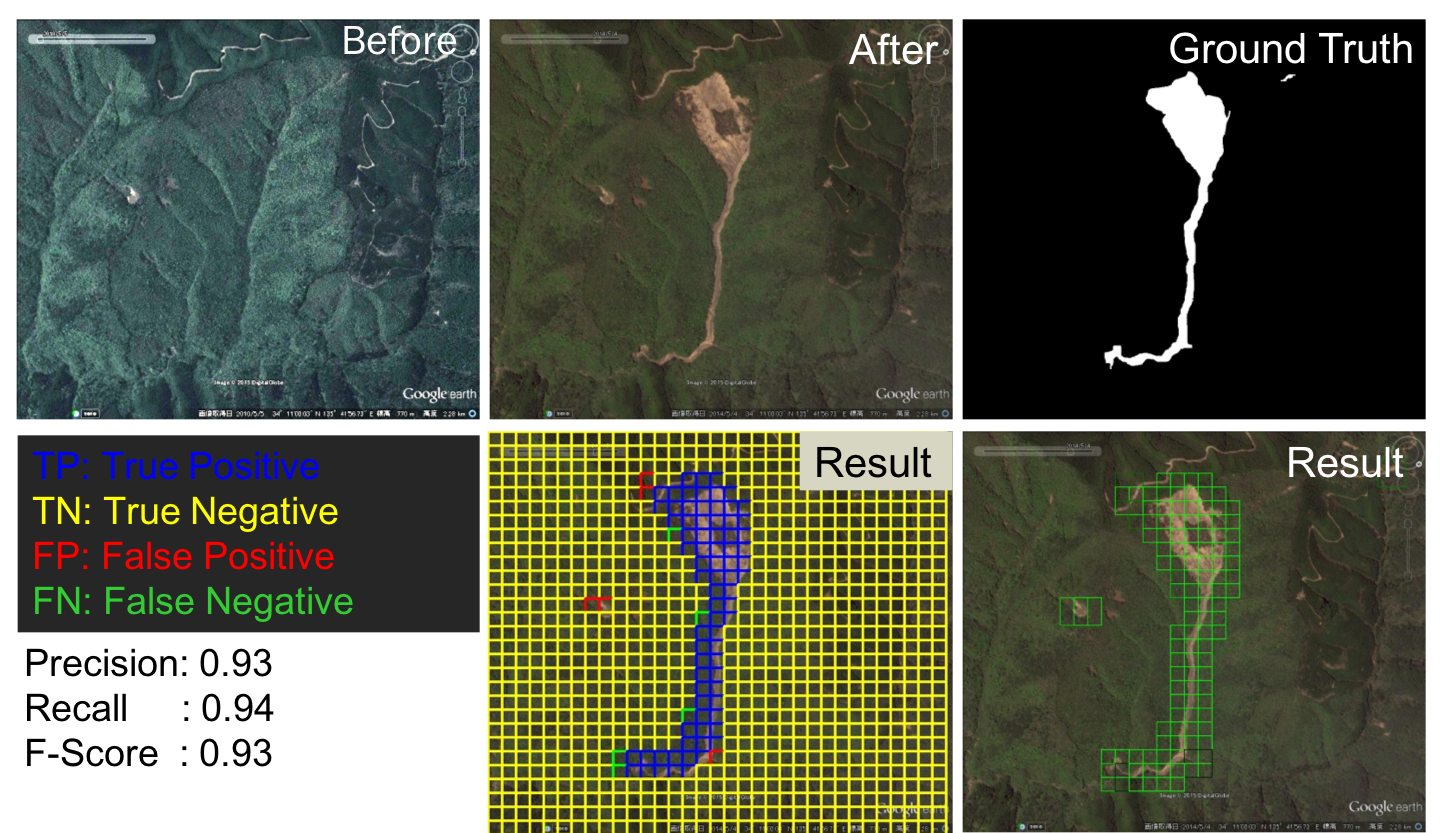