Object Manipulation Task Generation by Self-supervised Learning of Stationary Layouts
We propose a method for generating the operations to reconstruct a stationary object’s layout from an input image that has a non-stationary layout. The proposed method is an encoder-decoder–type network with special layers for estimating a list of operations which includes the operation type, object class, and position. The network can trained by a self-supervised manner. From an experiment of the operation generation using real images, it is confirmed that the our method have enabled generating the operations that change the object’s layout in an input scene to a stationary layout in real time.
Task-oriented Function Detection based on an operational task
We propose a novel representation to describe functions of an object, Task-oriented Function, which takes the place of Affordance in the field of Robotics Vision. We also propose a CNN-based network to detect Task-oriented Function. This network takes as input an operational task as well as a RGB image and assign each pixel to an appropriate label to every task. Because the outputs from the network differ depending on tasks, Task-oriented Function makes it possible to describe a variety of ways to use an object. We introduce a new dataset for Task-oriented Function, which contains about 1200 RGB images and 6000 annotations assuming five tasks. Our proposed method reached 0.80 mean IOU in our dataset.
Tactile Logging: A method of describing the operation history on an object surface based on human motion analysis
We propose a method to analyze a demonstration of a tool operated by a human and shot as an RGB-D movie. The proposed method estimates the interaction that occurs with the object while tracking the human pose and the three-dimensional position and attitude of the object subject to operation. This result is recorded as a time-series usage history (tactile log) onthe surface of the 3D model of the object. The tactile log is a new data expression for manifesting the ideal method of using the object, and it can be used for generating operations of gripping and handling “natural” tools by robot arms.
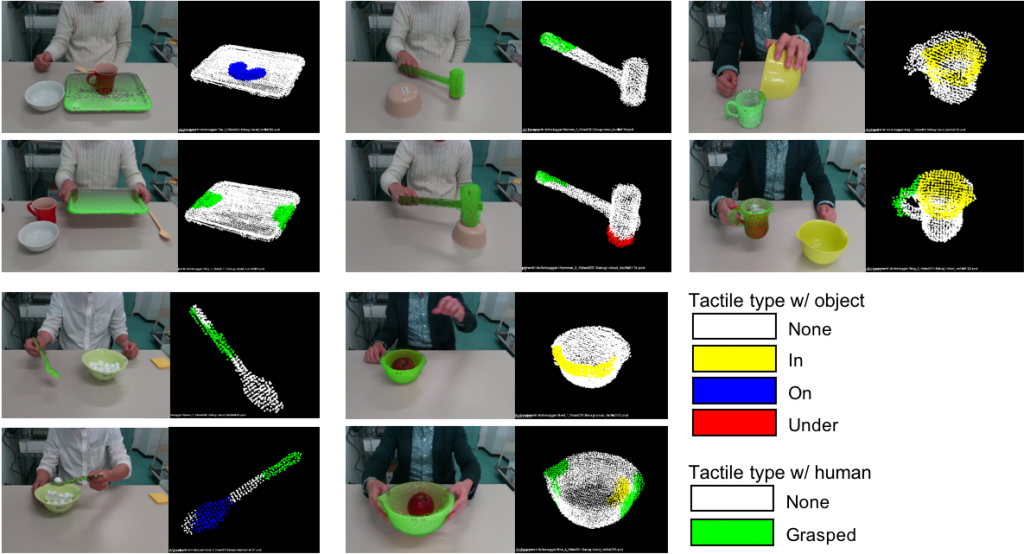
6-degree-of-freedom attitude estimation of similar-shaped objects focusing on the spatial arrangement of functional attributes
We propose a 6-degree-of-freedom attitude estimation method that can be operated even if the same 3D model as the object does not exist. Even if the design of tools in the same category is different, the arrangement of the roles (function attributes) of each part is considered to be common. In the proposed method, this is used as a means for attitude estimation. We confirmed that the reliability of attitude estimation improves by simultaneously optimizing consistency between arrangements of functional attributes and consistency between shapes. In actual use, this has the advantage that if just one 3D model of an object of a target category is associated with a function attribute or a grasping method, it is possible to handle an actual object just as it is, eliminating the necessity to prepare model data for each object.
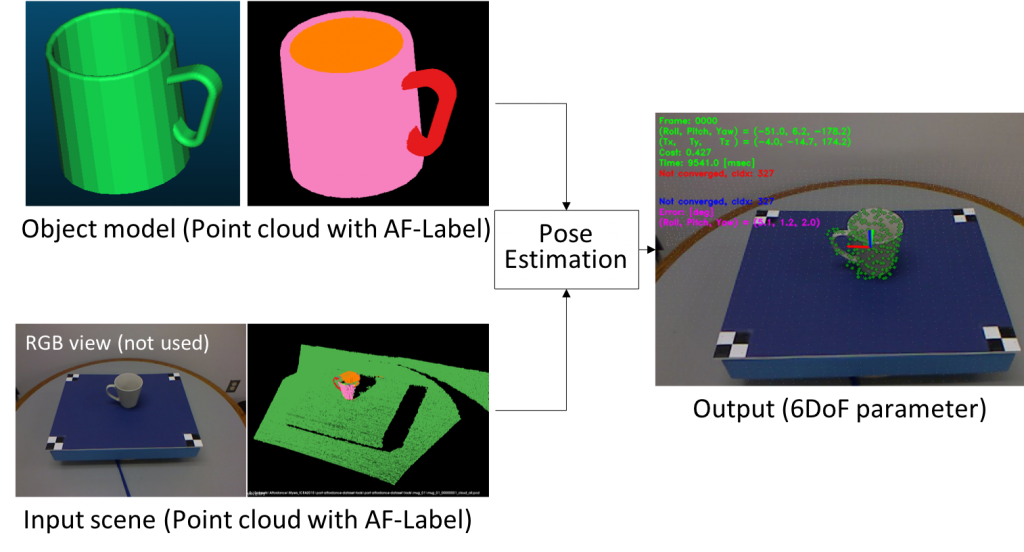
(Input:PointCloud with function attributes,Output:Posture transformation parameters)