Diverse Plausible 360-Degree Image Outpainting for Efficient 3DCG Background Creation
※Accepted to CVPR2022 : Arxiv , OSS
In 3DCG creation, 360-degree images are used as background images representing the entire surroundings to create scenes efficiently. Our research addresses the problem of generating a 360-degree image by using a single normal-angle image as input and completing its surroundings. Our transformer-based method provides higher resolution and more natural-looking output images than prior methods’ results. Furthermore, the proposed method can output diverse result images for a single input, thus giving users many choices for their creation. In this way, this research aims to support users in efficient 3DCG creation with originality.
Fast Soft Color Segmentation
※Accepted to CVPR2020 : Arxiv , OSS
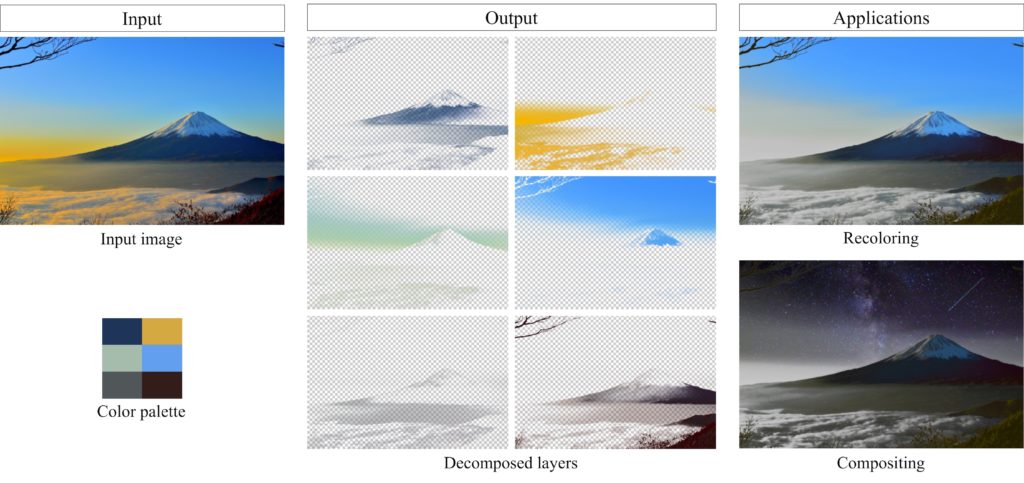
Visual Attribute Manipulation Using Natural Language Commands
In this paper, a novel setting is tackled in which a neural network generates object images with transferred attributes, by conditioning on natural language. Conventional methods for object image transformation have been known to bridge the gap between visual features by using an intermediate space of visual attributes. This paper builds on this approach and finds an algorithm to precisely extract information from natural language commands, completing this image translation model. The effectiveness of our information extraction model is experimented, with additional tests to see if the change in visual attributes is correctly seen in the image.
Graph Convolutional Neural Networks on superpixels for segmentation
A disadvantage of image domain segmentation using CNN is that spatial information is lost due to down-sampling by the pooling layer, and domain segmentation accuracy in the vicinity of object contours is reduced. Therefore, we proposed a graph convolution on superpixels as a different approach to prevent loss of information by pooling. In addition, we proposed a Dilated Graph Convolution, which extends the receptive field more effectively as an extension of the graph convolution. In a domain segmentation task using the HKU-IS data set, the proposed method outperformed a conventional CNN with the same configuration.
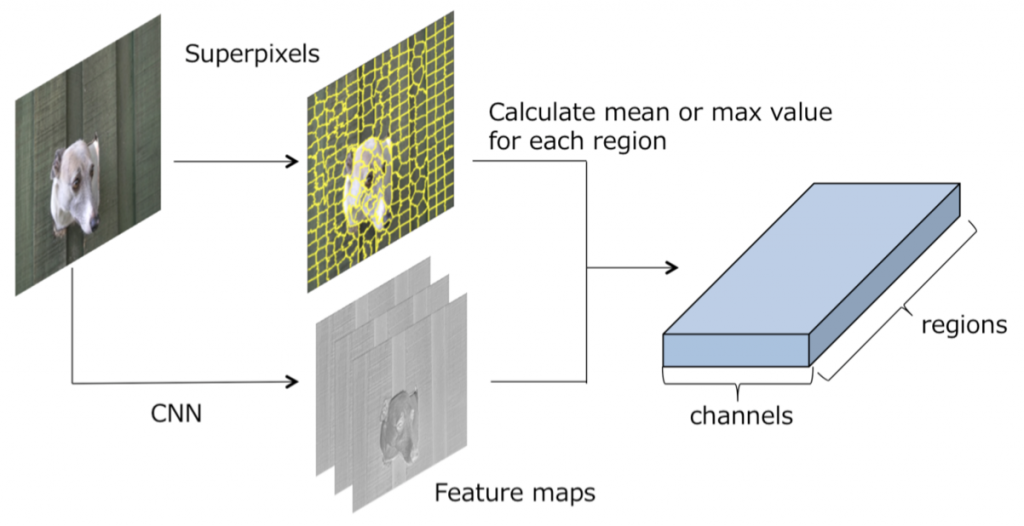
Saliency map generation in image discrimination using a CNN classifier
In general, when an image is input to a CNN and a specific output obtained, it is difficult to explain why such an output was obtained. In this study, we propose a saliency map generation method given by applying a Generative Adversarial Networks framework. In this system, learning takes place while two neural networks are made to compete. The first network learns to perform image identification. The second network learns to create an image that – if an image is input to the first network and can be successfully identified – is similar to this image but outputs an incorrect result when input into to the first network. For the second network to efficiently generate such an image, it suffices for the network to generate an image in which an image area important in the image identification of the first network is significantly changed. Such learning can be regarded as a saliency map, since it is possible to explicitly output an image area important in image identification.
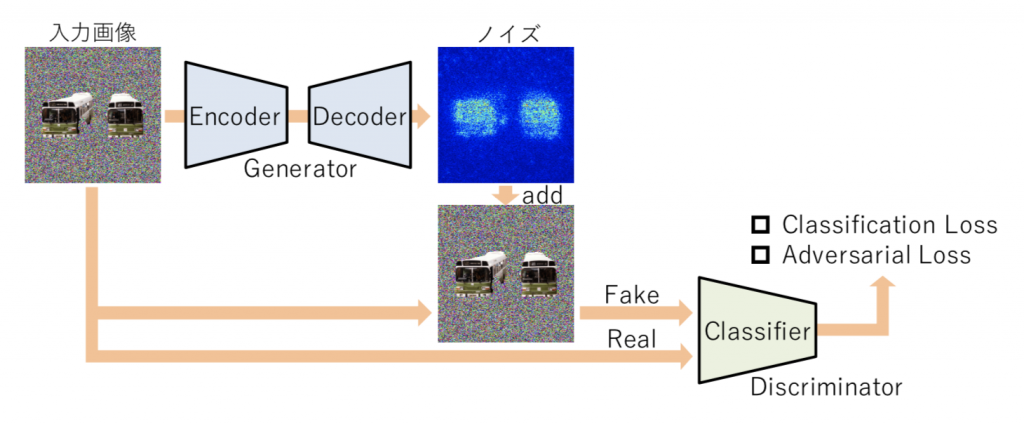
Simultaneous execution of color adjustment and image completion by GAN
In this study, we propose a method of image completion while performing color adjustment in consideration of context in order to solve the problem of natural paste synthesis by color adjustment and image completion. In order to make the inserted object image explicitly appear in the completion area, we use CNN and Generic Adversarial Networks (GAN) for completion in consideration of context, and extract features related to the context from the entire background image. Furthermore, color adjustment taking context into consideration is carried out using the context features not only for image completion but also for color adjustment. In this way, a network is realized that simultaneously solves the problems of color adjustment and image completion.
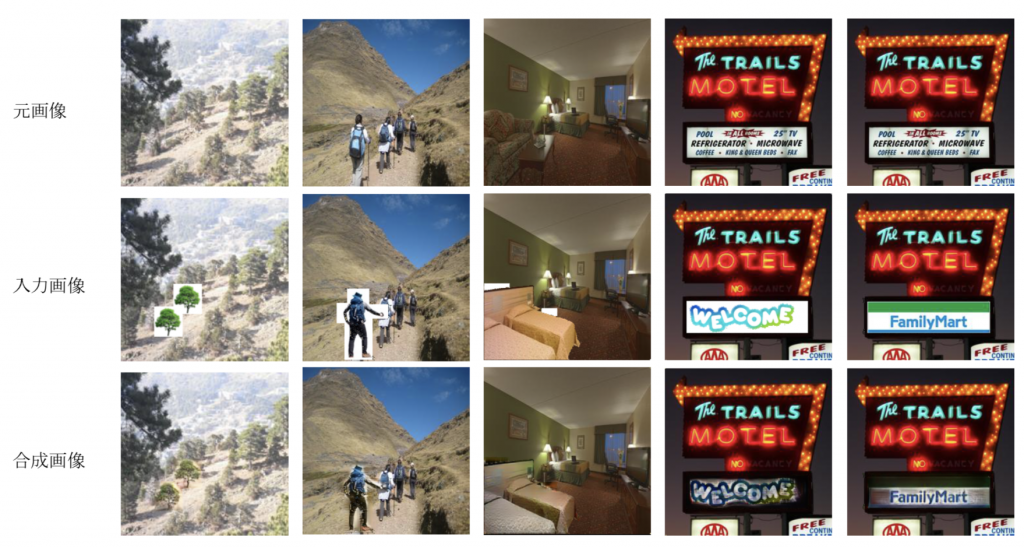
Image Completion of 360-Degree Images by cGAN
This work proposes the novel problem setting that by using a known area from the 360-degree image as an input, the remainder of the image can be completed with the GANs. To do so, we propose the approach of two-stage generation using network architecture with series-parallel dilated convolution layers. Moreover, we present how to rearrange images for data augmentation, simplify the problem, and make inputs for training the 2nd stage generator. Our experiments show that these methods generate the distortion seen in 360-degree images in the outlines of buildings and roads, and their boundaries are clearer than those of baseline methods. Furthermore, we discuss and clarify the difficulty of our proposed problem. Our work is the first step towards GANs predicting an unseen area within a 360-degree space.
Image control after imaging by epsilon photography reconstruction using compression sensing
Conventionally, the photographer must select many parameters on the camera at the time of photographing. Light field imaging has enabled image control after shooting with respect to focus position and shooting viewpoint, but resolution is low, specialist hardware is required, and a completely flexible restoration of the focus position and aperture size is not possible. This study relates to technology to restore images taken with various parameters from ten images shot in succession using conventional cameras with parameters such as focus position, aperture size, exposure time and ISO changed. For example, we completely reconstruct the high dynamic range focus-aperture stack using consecutively shot images with pre-set parameters as the input.
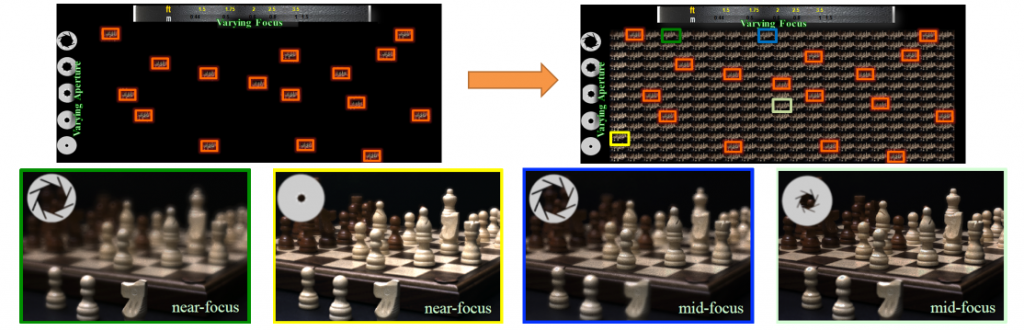